Abstract
Source localization based on measurements from a sensor array arise in many applications, e.g., mmWave wireless communications, Radar, Sonar, EEG, MEG, etc. among others. With the advent of compressed sensing (CS) and sparse signal recovery (SSR) algorithms, this problem has been revisited using this new tool. In this talk I will discuss a SSR algorithm based on Sparse Bayesian Learning (SBL) that employs a Gaussian scale mixture (GSM) prior. The Expectation-Maximization (SBL-EM) based inference algorithm will be examined and interpreted using a beamforming framework. A contrast with the classical minimum power distortion-less response (MPDR) beamformer will be drawn and the benefits highlighted. An interesting finding is the explanation to the ability of SBL to deal with correlated sources. For a uniform linear array (ULA), the Toeplitz approximation property of SBL will be discussed and the potential benefits for a nested array demonstrated by locating more sources than sensors.
Bio
Bhaskar D. Rao received the B.Tech. degree in electronics and electrical communication engineering from the Indian Institute of Technology, Kharagpur, India, in 1979 and the M.S. and Ph.D. degrees from the University of Southern California, Los Angeles, in 1981 and 1983, respectively. Since 1983, he has been with the University of California at San Diego, La Jolla, where he is currently a Distinguished Professor in the Electrical and Computer Engineering department. He is the holder of the Ericsson endowed chair in Wireless Access Networks and was the Director of the Center for Wireless Communications (2008-2011). Prof. Rao’s interests are in the areas of digital signal processing, estimation theory, and optimization theory, with applications to digital communications, speech signal processing, and biomedical signal processing.
Prof. Rao was elected fellow of IEEE in 2000 for his contributions to the statistical analysis of subspace algorithms for harmonic retrieval. His work has received several paper awards; 2013 best paper award at the Fall 2013, IEEE Vehicular Technology Conference for the paper “Multicell Random Beamforming with CDF-based Scheduling: Exact Rate and Scaling Laws,” by Yichao Huang and Bhaskar D Rao, 2012 Signal Processing Society (SPS) best paper award for the paper “An Empirical Bayesian Strategy for Solving the Simultaneous Sparse Approximation Problem,” by David P. Wipf and Bhaskar D. Rao published in IEEE Transaction on Signal Processing, Volume: 55, No. 7, July 2007, 2008 Stephen O. Rice Prize paper award in the field of communication systems for the paper “Network Duality for Multiuser MIMO Beamforming Networks and Applications,” by B. Song, R. L. Cruz and B. D. Rao that appeared in the IEEE Transactions on Communications, Vol. 55, No. 3, March 2007, pp. 618 630. (http://www.comsoc.org/ awards/rice.html), among others. Prof. Rao is also the recipient of the 2016 IEEE Signal Processing Society Technical Achievement Award.
Prof. Rao has been a member of the Statistical Signal and Array Processing technical committee, the Signal Processing Theory and Methods technical committee, the Communications technical committee of the IEEE Signal Processing Society and was the chair of the Machine learning for Signal Processing technical committee (2019-2020).
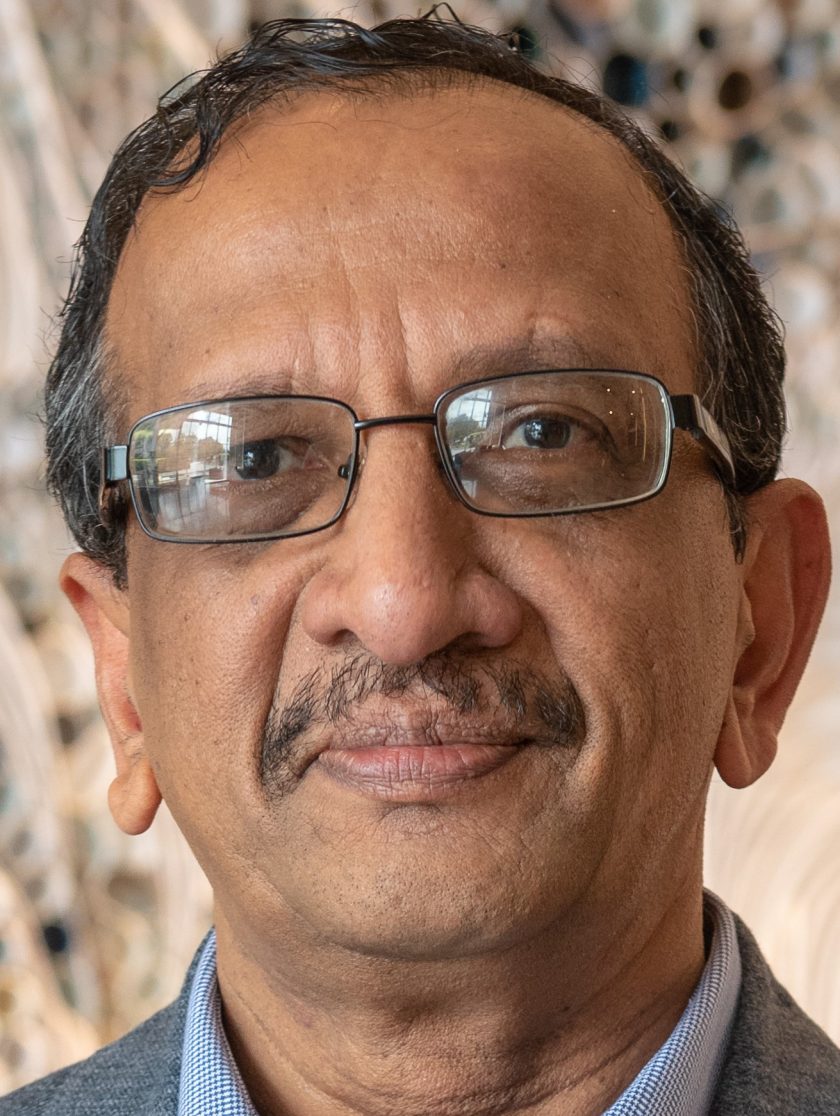