Abstract
Understanding the brain and its function is one of the grand challenges in modern science. The human brain has about 86 billion neurons, where each neuron talks to about a thousand other neurons; the complexity of this system far exceeds all other known natural or engineered systems. Within this massive, dynamic, and remarkably robust network arises sensation, action, thoughts, feelings, and the capacity to wonder what makes itself tick. (Yours is doing it right now!)
Advances in technology and infrastructure are rapidly increasing our ability to acquire and make sense of big, complex data measured from the brain in action. These data tax our analytic tools; fortunately, even very high-dimensional dynamic systems often exhibit low-dimensional coherent patterns. I will talk about a few of my recent projects that leverage this inherent sparsity in neural data to build parsimonious models, especially models that may interface well with closed-loop engineering design.
Biography
Bing Brunton is a Washington Research Foundation Innovation Assistant Professor in Neuroengineering at the University of Washington (UW) Department of Biology. She is also a Data Science Fellow of the UW eScience Institute and a faculty member of the Graduate Program in Neuroscience. She holds a B.S. in Biology from Caltech and a Ph.D. in Neuroscience from Princeton. Her research interests combine data-driven analytic techniques and ideas from dynamical systems to understand big, complex data measuring neural activity.
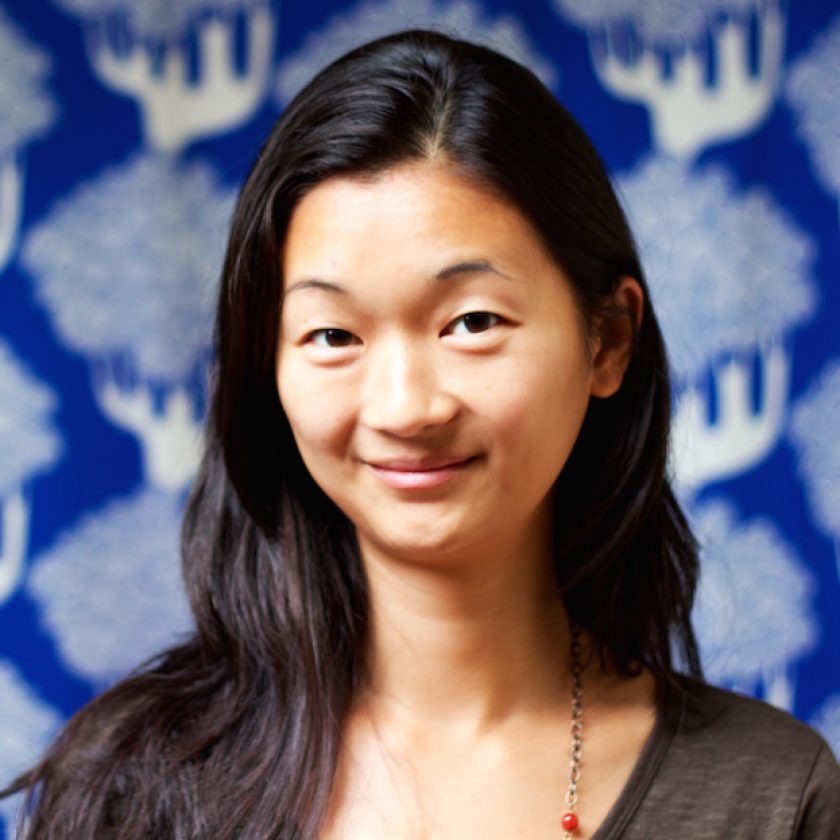