Abstract
Approximating high-dimensional functionals with low-dimensional models is a central issue of machine learning, image processing, physics and mathematics. Deep convolutional networks are able to approximate such functionals over a wide range of applications. This talk shows that these computational architectures take advantage of scale separation, symmetries and sparse representations. We introduce simplified architectures which can be analyzed mathematically. Scale separation is performed with wavelets and scale interactions are captured through phase coherence. Pattern structures are captured by sparse coding in dictionaries. We show applications to image classification and generation, as well as regression of quantum molecular energies and modelization of turbulent flows.
Bio
Dr. Stéphane Mallat is known for his fundamental work in wavelet theory, with major impact in machine learning, signal processing, music synthesis, harmonic analysis, and image segmentation. He received a Ph.D. from the University of Pennsylvania in 1988, after which he became a Professor at the Courant Institute of Mathematical Sciences. In 1995, he became Professor of Applied Mathematics at Ecole Polytechnique, Paris and Department Chair in 2001. From 2012 to 2017 he was Professor in the Computer Science Department of Ecole Normale Supérieure, in Paris. Since 2017, he has held the “Data Sciences” chair at the Collège de France. He is a member of the French Academy of Sciences, a foreign member of the US National Academy of Engineering, an IEEE Fellow and an EUSIPCO Fellow. In 1997, he received the Outstanding Achievement Award from the SPIE Society and was a plenary lecturer at the International Congress of Mathematicians in 1998. He also received the 2004 European IST Grand prize, the 2004 INIST-CNRS prize for most cited French researcher in engineering and computer science, the 2007 EADS grand prize of the French Academy of Sciences, the 2013 Innovation medal of the CNRS, and the 2015 IEEE Signal Processing best sustaining paper award.
Additional Information
Dr. Stéphane Mallat will also be giving a lecture at Lytle Lecture later in the day; Tuesday, Dec. 3, 3:30 to 5:30 p.m. (doors open at 3:00 p.m.), Paul G. Allen Center Atrium. That Lecture is titled “Mathematical Mysteries of Deep Neural Networks”. Lytle Lecture is free and open to the public, click the link below to view the Abstract for Dr. Stéphane Mallat’s talk at Lytle Lecture.
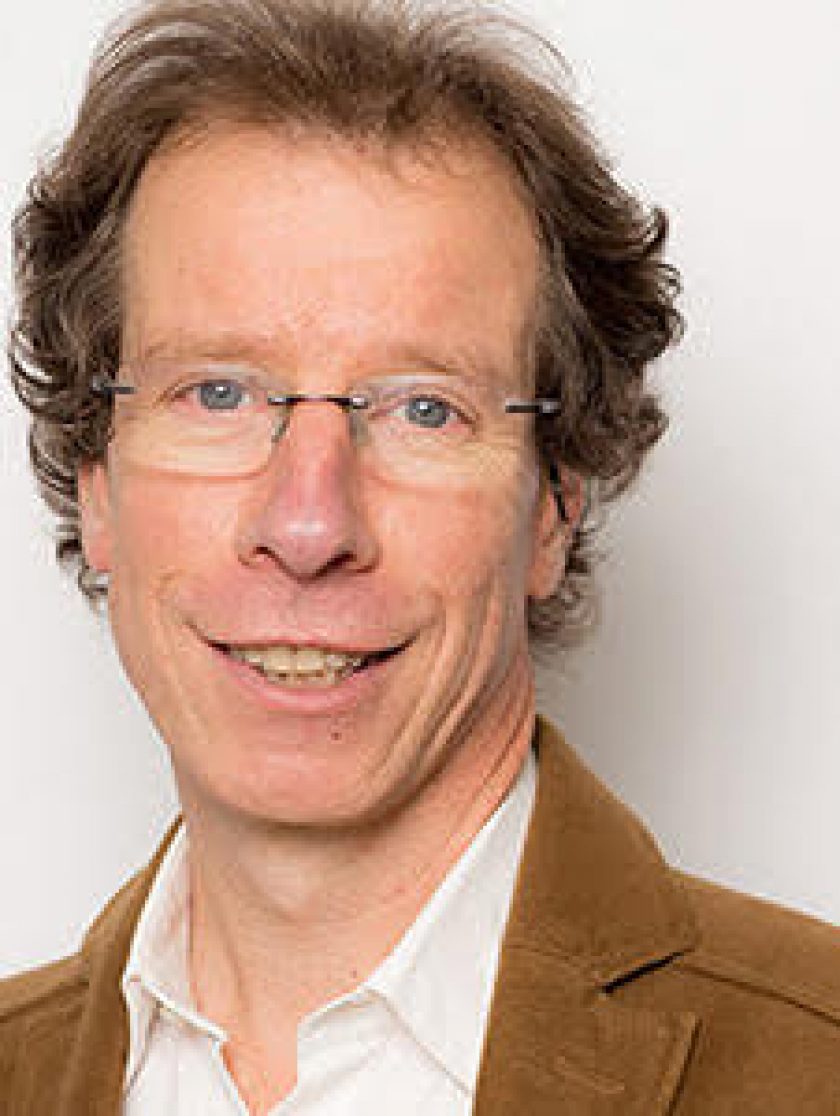