Abstract
Deep neural networks obtain impressive results for image, sound and language recognition or to address complex problems in physics. They are partly responsible for the renewal of artificial intelligence. Yet, we do not understand why they can work so well and why they sometimes fail, which raises many problems of robustness and explainability.
Recognizing or classifying data amounts to approximate phenomena which depend on a very large number of variables. The combinatorial explosion of possibilities makes it potentially impossible to solve. One can learn from data only if the problem is highly structured. Deep neural networks appear to take advantage of the existence of such structures, whose nature seem to be similar across a wide range of applications. Understanding this “architecture of complexity” involves many branches of mathematics and is related to open questions in physics. I will discuss some approaches and show applications.
Bio
Dr. Stéphane Mallat is known for his fundamental work in wavelet theory, with major impact in machine learning, signal processing, music synthesis, harmonic analysis, and image segmentation. He received a Ph.D. from the University of Pennsylvania in 1988, after which he became a Professor at the Courant Institute of Mathematical Sciences. In 1995, he became Professor of Applied Mathematics at Ecole Polytechnique, Paris and Department Chair in 2001. From 2012 to 2017 he was Professor in the Computer Science Department of Ecole Normale Supérieure, in Paris. Since 2017, he has held the “Data Sciences” chair at the Collège de France. He is a member of the French Academy of Sciences, a foreign member of the US National Academy of Engineering, an IEEE Fellow and an EUSIPCO Fellow. In 1997, he received the Outstanding Achievement Award from the SPIE Society and was a plenary lecturer at the International Congress of Mathematicians in 1998. He also received the 2004 European IST Grand prize, the 2004 INIST-CNRS prize for most cited French researcher in engineering and computer science, the 2007 EADS grand prize of the French Academy of Sciences, the 2013 Innovation medal of the CNRS, and the 2015 IEEE Signal Processing best sustaining paper award.
Additional Information
To reserve your seat for this event, please click here.
Dr. Stéphane Mallat will also be giving a lecture at the Colloquium Series earlier in the day; Tuesday, Dec. 3, 10:30 to 11:30 a.m., 105 ECE Building, University of Washington. That Lecture is titled “Interpretable Deep Networks for Classification, Generation and Physics”. The Colloquium Series is free and open to the public. Please click the following link to view the Abstract for Dr. Mallat’s talk at the Colloquium Series.
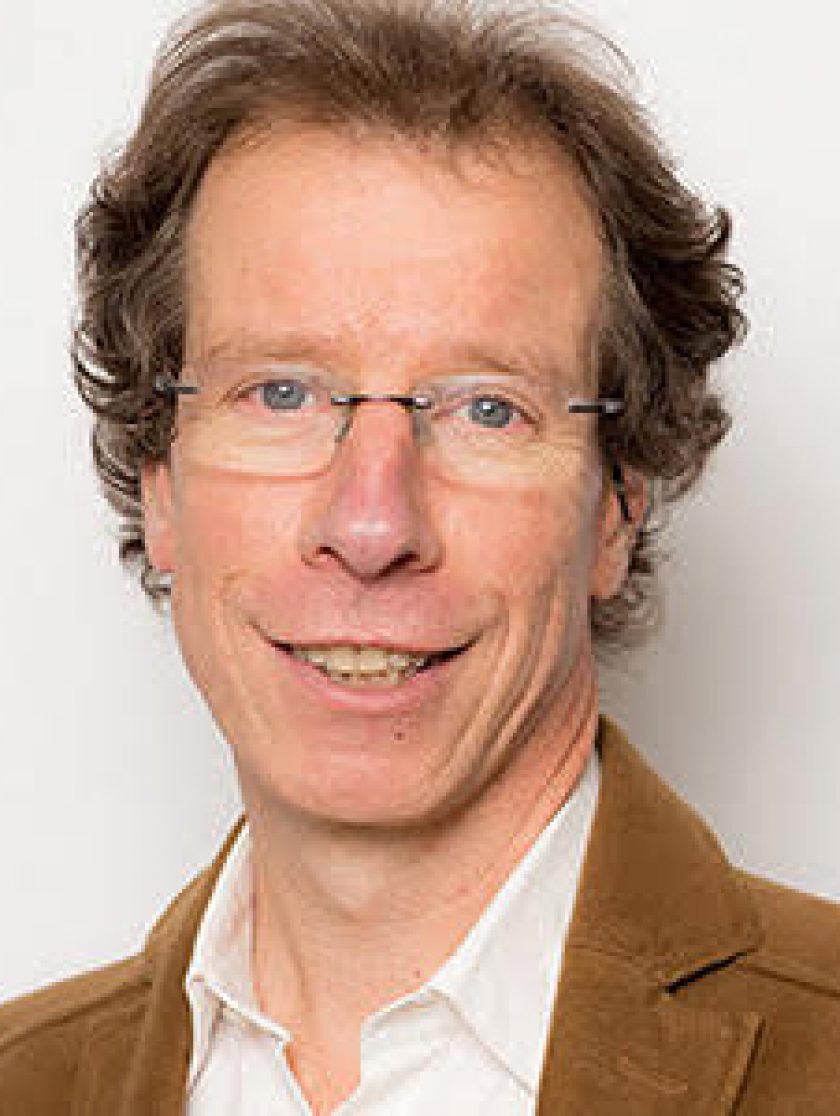