Story by Wayne Gillam | UW ECE News
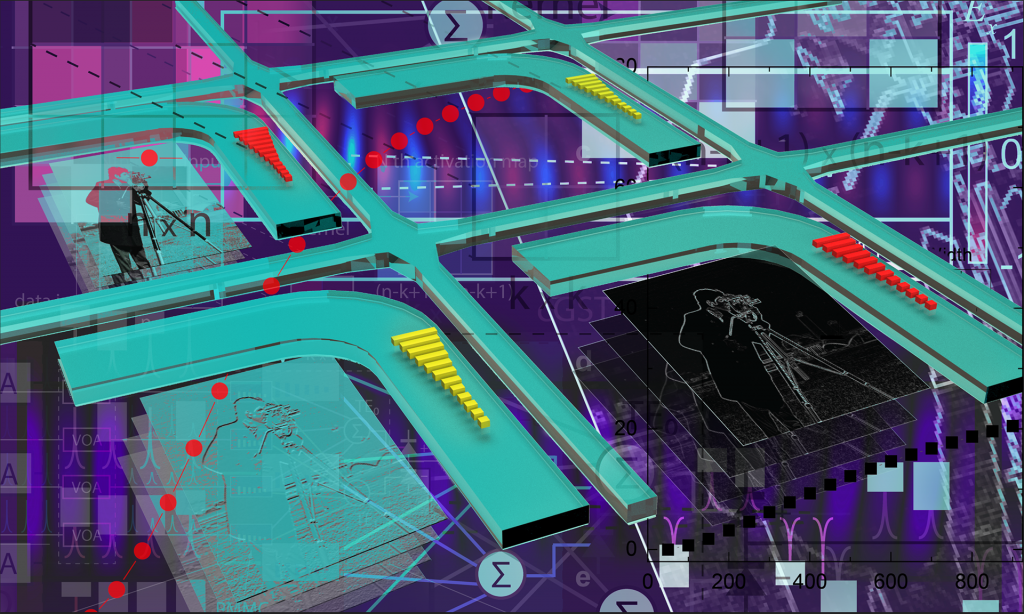
A UW ECE research team led by associate professor Mo Li, in collaboration with researchers at the University of Maryland, has developed an optical computing system that could contribute toward speeding up AI and machine learning — and thus the performance of our favorite software applications — while reducing associated energy and environmental costs. The team is also among the first in the world to use phase-change material in optical computing to enable image recognition by an artificial neural network, a benchmark test of a neural network’s computing speed and precision. (Illustration by Ryan Hoover)
Artificial intelligence (AI) and machine learning are already an integral part of our everyday lives online, although many people may not yet realize that fact. For example, search engines such as Google are facilitated by intelligent ranking algorithms, video streaming services such as Netflix use machine learning to personalize movie recommendations, and cloud computing data centers use AI and machine learning to facilitate a wide array of services. The demands for AI are many, varied and complex. As those demands continue to grow, so does the need to speed up AI performance and find ways to reduce its energy consumption. On a large scale, energy costs associated with AI and machine learning can be staggering. For example, cloud computing data centers currently use an estimated 200 terawatt hours per year — more than a small country — and that energy consumption is forecasted to grow exponentially in coming years with serious environmental consequences.
Now, a research team led by associate professor Mo Li at the University of Washington Department of Electrical & Computer Engineering (UW ECE), in collaboration with researchers at the University of Maryland, has come up with a system that could contribute toward speeding up AI while reducing associated energy and environmental costs. In a paper published January 4, 2021, in Nature Communications the team described an optical computing core prototype that uses phase-change material (a substance similar to what CD-ROMs and DVDs use to record information). Their system is fast, energy efficient and capable of accelerating neural networks used in AI and machine learning. The technology is also scalable and directly applicable to cloud computing, which uses AI and machine learning to drive popular software applications people use everyday, such as search engines, streaming video, and a multitude of apps for phones, desktop computers and other devices.
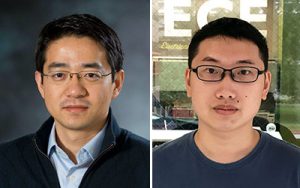
UW ECE associate professor Mo Li (left) and UW ECE graduate student Changming Wu (right) led the research team that built the optical computing system prototype. Their system uses phase-change material (a substance similar to what CD-ROMs and DVDs use to record information) to facilitate AI computing speed and energy efficiency.
“The hardware we developed is optimized to run algorithms of an artificial neural network, which is really a backbone algorithm for AI and machine learning,” Li said. “This research advance will make AI centers and cloud computing more energy efficient and run much faster.”
The team is among the first in the world to use phase-change material in optical computing to enable image recognition by an artificial neural network. Recognizing an image in a photo is something that is easy for humans to do, but it is computationally demanding for AI. Because image recognition is computation-heavy, it is considered a benchmark test of a neural network’s computing speed and precision. The team demonstrated that their optical computing core, running an artificial neural network, could easily pass this test.
“Optical computing first appeared as a concept in the 1980s, but then it faded in the shadow of microelectronics,” said lead author Changming Wu, who is an electrical and computer engineering graduate student working in Li’s lab. “Now, because of the end of Moore’s law [the observation that the number of transistors in a dense, integrated circuit doubles about every two years], advances in integrated photonics, and the demands of AI computing, it has been revamped. That’s very exciting.”
Speeding up hardware and software performance
Optical computing is fast because it uses light generated by lasers — instead of the much slower electricity used in traditional digital electronics — to transmit information at mind-boggling speeds. The prototype the research team developed was designed to accelerate computational speed of an artificial neural network, and that computing speed is measured in billions and trillions of operations per second. According to Li, future iterations of their device hold the potential to go even faster.
“This is an early prototype, and we are not using the highest speed possible with optics yet,” Li said. “Future generations show the promise of going at least an order of magnitude faster.”
In the eventual real-world application of this technology, that means any software powered by optical computing through the cloud — such as search engines, video streaming and cloud-enabled devices — would run faster as well, improving performance.
Increased energy efficiency
Li’s research team took their prototype one step further by using phase-change material to store data and perform computing operations by detecting the light transmitted through the material. Unlike transistors used in digital electronics that require a steady voltage to represent and hold the zeros and ones used in binary computing, phase-change material doesn’t require any energy at all to hold this information. Just like in a CD or DVD, when phase-change material is heated precisely by lasers it switches between a crystalline and an amorphous state. The material then holds that state or “phase,” along with the information the phase represents (a zero or one), until it is heated again by the laser.
“There are other competing schemes to construct optical neural networks, but we think using phase-changing material has a unique advantage in terms of energy efficiency because the data is encoding in a non-volatile way, meaning that the device, using phase-changing material, does not consume a constant amount of power to store the data,” Li explained. “Once the data is written there, it’s always there. You don’t have to supply power to keep it in place.”
This energy-savings matters, in that when it is multiplied by millions of computer servers at thousands of data centers around the world, the reduction in energy consumption and environmental impact will be significant.
Optimizing and scaling up for the real world
The team further enhanced the phase-change material used in their optical computing core by patterning the material into nanostructures. These microscopic constructions improve the material’s endurance and stability, it’s contrast (the ability to distinguish between zero and one in binary code), and enable greater computational capacity and precision. Li’s research team also fully integrated phase-change material into the prototype’s optical computing core.
“Here, we are doing everything we can to integrate optics,” Wu said. “We put the phase-change material on top of a waveguide, which is a tiny little wire we carve on the silicon chip that guides light. You can think of it as an electrical wire for light, or as an optical fiber carved on the chip.”
Li’s research team asserts that the method they developed is one of the most scalable approaches to optical computing technologies currently available, eventually applicable to large systems such as networked cloud computing servers at data centers around the world.
“Our design architecture is scalable to a much, much larger network that can handle challenging artificial intelligence tasks ranging from large, high-resolution image recognition to video processing and video image recognition,” Li said. “Our scheme is the most promising one, we believe, that’s scalable to that level. Of course, that will take industrial-scale semiconductor manufacturing. Our scheme and the material that makes up the prototype are all very compatible with semiconductor foundry processes.”
The future is light
Looking forward, Li said he could envision optical computing devices, such as the one his team developed, providing a further boost to the computational performance of current technology and enabling the next generation of artificial intelligence. To move in that direction, the next steps for his research team will be to scale up the prototype they built by working closely with UW ECE associate professor Arka Majumdar and assistant professor Sajjad Moazeni, experts in large-scale integrated photonics and microelectronics.
And after the technology is scaled up sufficiently, it will lend itself to future integration with energy-hungry data centers, speeding up the performance of software applications facilitated by cloud computing and driving down energy demands.
“Nowadays in data centers, the computers are already connected by optical fibers. This provides the ultra-high bandwidth communication that is really needed,” Li said. “So, it’s logical to perform optical computing in such a setting because fiber optics infrastructure is already done. It’s exciting, and I think the time is about right for optical computing to emerge again.”
The research described in this article is supported by the Office of Naval Research through a Department of Defense Multidisciplinary University Research Initiative (MURI) program.