By Wayne Gillam | UW ECE News
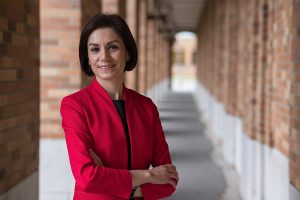
UW ECE Professor and Associate Chair for Research Maryam Fazel. Photo by Ryan Hoover
Most automated technologies that we use, enjoy and rely on today are built on applications driven by data science and machine learning. Internet search engines such as Google, movie and music recommendations systems embedded in popular entertainment applications such as Netflix and Spotify, and even traffic signals and airline routes are guided by mathematical algorithms working steadily behind the scenes, making decisions and creating outcomes that help to determine the user’s overall experience.
But somewhere in the race to develop, market and deploy these fast-changing technologies, the theoretical understanding of how underpinning algorithms function and interact with each other fell behind the ability to implement them, and this has led to unintended consequences. For example, search engines might show us only news that aligns with our current beliefs or expectations, essentially walling us off from information that could challenge or expand our point of view, or recommender systems might only serve up movies or music from a limited number of genres, based on our past choices. In some of the worst cases, these quirks in systems that depend on algorithms can cause serious problems, such as contributing to disinformation and extremism on social media. Algorithmic flaws can also have concerning impacts on transportation systems, robotics, online security, healthcare and other vital areas of the economy that have increasingly come to rely on automation and machine learning.
To help address these issues, UW ECE Professor and Associate Chair for Research Maryam Fazel, who holds the Moorthy Family Inspiration Career Development Professorship, is leading the Institute for Foundations of Data Science, or IFDS, which is a collaboration between the UW and the Universities of Wisconsin-Madison, California-Santa Cruz, and Chicago. The IFDS launched in September 2020 with a broad mandate to build a fundamental understanding of data science, and it is one of only two institutes nationwide funded by the National Science Foundation’s Transdisciplinary Research In Principles of Data Science (TRIPODS) Phase II grant. Through studying and developing the theoretical foundations of this fast-changing field, the IFDS aims to tackle complex algorithmic challenges at the root of these problems.
Over the past year, the IFDS has launched several collaborative research projects and educational programs within the UW and between its participating universities and affiliates. Following is a question-and-answer session with Fazel, which explains in more detail the importance of this Institute and its anticipated impact.
Can you briefly describe what the IFDS does?
The big goal of the IFDS is to produce robust, reliable, privacy-preserving, fair data science algorithms that can perform well in dynamic and complex environments. Each of these areas represents a huge challenge. At the IFDS, we are trying to address a little bit of all these challenges, working toward the goal of improving data science algorithms in substantive ways, so they operate more effectively.
Why is a theoretical understanding of data science algorithms important?
Because things can go wrong. Basically, this is the danger: people are using algorithms without really knowing how they work. You would think that in science and engineering until something is very well understood, it’s not going to be deployed. But in the area of machine learning and artificial intelligence, algorithms and models were deployed right away, before developing a firm understanding of how they work.
IFDS emphasizes the “foundations.” We need to deeply understand and come up with theoretical explanations to first understand how an algorithm works, and then, to fix problems, fix the issues that arise. Occasionally, something goes wrong with an algorithm, and nobody knows why. Having an understanding of the underlying system helps with addressing such issues.
Another reason why theory is important is that historically, big technological advances have been enabled by development of solid theory. For example, in the field of aerospace, before there was a successful landing on the moon, control theory developed a lot of the tools used to predict trajectories and design mechanisms within the spacecraft. Solid theoretical foundations have already been built for fields such as aerospace and communications. Our hope is that we can provide a similar foundation for data science, machine learning and artificial intelligence.
The IFDS brings together mathematicians, statisticians, computer scientists and engineers. Why did the Institute’s leadership choose this interdisciplinary approach?
Data science questions touch upon many different fields, so to find effective solutions to the challenges we’re working on, you need to bring teams of experts together who have different expertise, different points of view and get them to work together. Once you put together, for example, the math, statistics, computer science and engineering perspectives, then there is a lot more hope to make progress. That is why we have formed collaborative teams across four departments, both within the UW and between our partner universities and affiliates.
In total, we have four partner universities at the IFDS, 17 total faculty investigators, and six of those are at the UW. We also have a large and growing list of faculty and local affiliates from the UW, Microsoft Research, Meta Research and Amazon. In addition, over the past year, we have provided partial research funding for 14 graduate students and three postdoctoral scholars that are each co-mentored in at least two different fields.
Can you describe some of the projects you are working on at the IFDS?
Sure. In a project that I’m working on with Professor Mehran Mesbahi in the William E. Boeing Aeronautics and Astronautics Department at the UW, we are aiming to provide a theoretical basis for a common method used in reinforcement learning that, for example, is used in autonomous driving, playing games and computer programs such as AlphaGo. Ultimately, we hope to help explain and improve reinforcement learning algorithms more broadly.
In another interesting IFDS project, I am collaborating with Professor Kevin Jamieson from the Paul G. Allen School of Computer Science & Engineering, Professor Lalit Jain from the Foster School of Business and two IFDS students. The project focuses on closed-loop data collection, aiming to make the same inferences with less data, by collecting it in a smart way. We are designing new selective sampling algorithms that use fewer data labels. This is important because labeling data can be expensive.
I am also excited about a new project with UW ECE Professor Lillian Ratliff and Professor Dmitriy Drusvyatskiy from the UW Department of Mathematics, which involves two IFDS students. This team is studying algorithms that interact with humans and where population data reacts to the actions of competing decision makers, for example, loan decisions made by different banks and admission decisions of different universities. The team is using mathematical tools from game theory and optimization to design algorithms that systematically take into account feedback effects and the fact that people react strategically to algorithmic decisions.
What sort of impact do you anticipate the IFDS will have on everyday life?
The downstream impacts of this work on people’s lives can be broad and far-reaching. Algorithms are frequently deployed for data processing, automated reasoning and decision-making in computer applications, online security systems, transportation systems, online financial transactions — pretty much everywhere. So, the progress we make in developing theory and improving our understanding of how algorithms work will have a deep, lasting and positive impact on technologies people depend on worldwide.
Is there anything else you would like people to know about the IFDS?
Education is interwoven into our research efforts. All our projects involve university students in some capacity, and we are offering several summer programs for university and high school students. For example, we have a series of upcoming events at the UW in Summer 2022. AI4All@UW is a two-week summer workshop for high school students with a focus on students with disabilities. It is organized by Anat Caspi, who is the director of the Taskar Center for Accessible Technology. The PIMS-IFDS-NSF Summer School on Optimal Transport is a two-week program for university students, led by Professor Soumik Pal in the UW Department of Mathematics, which the IFDS is helping to organize. We’ll also be holding an IFDS Research Workshop on Distributional Robustness in Statistical Learning this summer and an annual meeting and research showcase. Details about these events, workshops and programs will be forthcoming on the IFDS website.
Overall, we’re off to a great start. Many of the collaborations we initiated this year are already proving to be quite productive. I am really looking forward to the progress we will be making over the next few years in data science research and education.
For more information about the IFDS, contact Maryam Fazel.