Article by Wayne Gillam, Photos by Ryan Hoover | UW ECE News
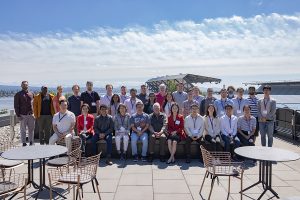
Participants in the Institute for Foundations of Data Science’s 2022 IFDS Workshop on Distributional Robustness in Data Science. The event was held in early August on the UW campus at the Bill & Melinda Gates Center for Computer Science & Engineering.
Artificial intelligence, machine learning and the mathematical algorithms that underpin these technologies affect our lives every day. For example, recommender systems within Netflix and Spotify learn our interests and serve up movies and music accordingly, natural language processing within assistants such as Siri and Alexa help to interpret our vocal commands, and facial recognition technology helps to find and sort our photos on social media. AI and machine learning are also used in a wide range of areas with important impacts to individuals and communities, such as health care, banking and online security.
However, many of the algorithms within AI and machine learning applications in use today are not as resilient to shifts in data and changes in the operating environment as they could be. Typical algorithms often assume that the data they work with follows a fixed distribution. But in reality, this data distribution is constantly changing and shifting because of a variety of factors, such as algorithms being deployed in a complex environment that changes over time, underreported or missing data, or even human responses to decisions an algorithm makes. These real-world complications can result in unexpected and negative outcomes such as errors in decisions and algorithmic biases, which can cause some population groups to be favored over others.
“Machine learning algorithms are used everywhere, and they’re used in settings without full knowledge from a theoretical standpoint of how they will work.” — Maryam Fazel, UW ECE Moorthy Family Professor and IFDS Director
A big question that researchers are grappling with today is whether algorithms could be designed to adapt to such changes and handle the corresponding effects with minimal disruption. Could major failures and unintended consequences that arise when data distribution changes be prevented? And if so, how well could this be accomplished? Finding satisfactory answers to these questions could help make AI and machine learning algorithms more reliable and adaptable to the real world, preventing many of the thorny issues described above.
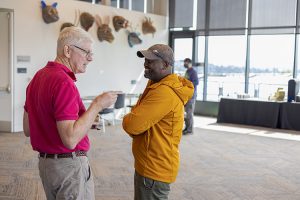
IFDS Wisconsin site director Stephen Wright (left) and Columbia University professor Samory Kpotufe (right) having a lively discussion at the workshop. “Creating a sense of community is so important in an endeavor like IFDS,” Wright said. “It brings together people with different perspectives and creates the right conditions for interesting new research to emerge.”
The Institute for Foundations of Data Science recently brought mathematicians, statisticians, computer scientists and other data science experts to the University of Washington campus to discuss ways of addressing these questions at the IFDS Workshop on Distributional Robustness in Data Science, which was held in early August at the Bill & Melinda Gates Center for Computer Science & Engineering. As its name suggests, the workshop focused on exploring “distributional robustness.” This is a promising framework and research area in data science aimed at addressing complex shifts and changes in data, which are fielded by automated devices and processes such as the algorithms used in AI and machine learning.
“It is a key challenge in machine-learning data science and AI nowadays because machine learning algorithms are used everywhere, and they’re used in settings without full knowledge from a theoretical standpoint of how they will work,” said UW ECE Moorthy Family Professor Maryam Fazel, who is the director of the IFDS and a member of the workshop’s steering committee. “From the IFDS’ perspective, this workshop directly ties into our research mission, and it is well aligned with three of four core themes we are exploring at the Institute — data robustness, closed-loop data science and ethics in algorithms. We are very excited about the new ideas that are inspired by the workshop.”
“Distributional robustness presents challenges but also opportunities to think about both purely technical aspects and socio-technical aspects of data science” — Professor Zaid Harchaoui, UW Department of Statistics, IFDS workshop Program Chair
The IFDS is funded by the National Science Foundation, and it is a collaboration between the UW and the Universities of Wisconsin-Madison, California Santa Cruz, and Chicago. The mission of the Institute is to develop a principled approach to the analysis of complex, automated, decision-making algorithms and ever larger and potentially biased data sets that play an increasingly important role in industry, government and academia.
“Workshops are a vital annual activity of the IFDS because they give us the opportunity to focus on an area of particular interest across the Institute and to engage the wider data science community by inviting experts to speak,” said Stephen Wright, who is a computer science professor at the University of Wisconsin-Madison, IFDS’ Wisconsin site director and also a member of the workshop’s steering committee. “Creating a sense of community is so important in an endeavor like IFDS. It brings together people with different perspectives and creates the right conditions for interesting new research to emerge.”
New research collaborations, a unique educational experience
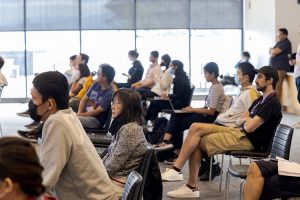
The workshop brought mathematicians, statisticians, computer scientists and other data science experts to the University of Washington campus. Attendees noted that they benefited from hearing how challenges in data science were being addressed from different points of view
Speakers and attendees came to the workshop from institutions of higher learning across the country, and UW ECE, the Paul G. Allen School of Computer Science & Engineering, the UW Department of Mathematics, and the UW Department of Statistics were all well-represented at the event. Invited speakers were from a wide range of academic disciplines and well over half of the invited speakers were female or from underrepresented minority groups. The talks covered theoretical, technical and socio-technical aspects of distributional robustness.
“Distributional robustness presents challenges but also opportunities to think about both purely technical aspects and socio-technical aspects of data science,” said Zaid Harchaoui, who is a professor in the UW Department of Statistics, an IFDS founding member and part of the Institute’s leadership, and the workshop’s program chair. “Many creative advances in the area of distributional robustness are made by young researchers, and the workshop program reflected this.” He added, “We announced the event across many research and education communities to reach out to a broad and diverse audience.”
“Bringing in experts to all speak about a central theme, especially in person, has a way of sparking conversations and research directions that would not have otherwise happened.” — Assistant Professor Kevin Jamieson, Paul G. Allen School of Computer Science & Engineering
Attendees noted that they benefited from hearing how challenges in data science were being addressed from different points of view. Several of the graduate students and postdoctoral researchers gave software demonstrations, which introduced new research tools to the broader group. The workshop itself provided a forum for discussion and starting new research collaborations, as well as a unique opportunity for participants to better understand distributional robustness from many different angles.
“The presence of so many experts gave me a wider perspective on the problem, particularly the statistical aspects,” Wright said. “The talks were all high quality. I was inspired to keep working on this topic of distributional robustness in close collaboration with IFDS postdocs and faculty.”
“Bringing in experts to all speak about a central theme, especially in person, has a way of sparking conversations and research directions that would not have otherwise happened,” said Kevin Jamieson, a workshop attendee, member of the IFDS leadership team and an assistant professor in the Paul G. Allen School of Computer Science & Engineering. “Students and I are already following up on ideas discussed at the workshop with other participants, and there is no telling what long-term research contributions and collaborations will result.”
Abstracts and full videos of all talks from this workshop can be found on the event website. Information about other Institute events and activities is available at the IFDS website.