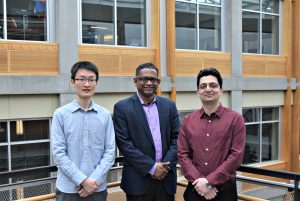
Graduate student Baicen Xiao, Professor and Chair Radha Poovendran and graduate student Hossein Hosseini.
University of Washington researchers have shown that Google’s new tool that uses machine learning to automatically analyze images can be defeated by adding noise.
Google recently released its Cloud Vision API to help developers to build applications that can quickly recognize objects, detect faces and identify and read texts contained within images. For any input image, the API also determines how likely it is that the image contains inappropriate contents, including adult, spoof, medical or violence contents.
In a new research paper, the UW electrical engineers and security expert team, consisting of doctoral students Hossein Hosseini and Baicen Xiao and Professor Radha Poovendran, demonstrated that the API can be deceived by adding a small amount of noise to images. After inputting the noisy image, the API outputs irrelevant labels, does not detect faces and fails to identify any text.
The same research team recently demonstrated the vulnerability of two other Google’s machine-learning-based platforms for detecting toxic comments and analyzing videos. They showed that toxic comment detection system Perspective can be easily deceived by typos, misspelling offensive words or adding unnecessary punctuation. They also showed that the Cloud Vision API can be deceived by inserting a photograph into a video at a very low rate.
“Machine learning systems are generally designed to yield the best performance in benign settings. But in real-world applications, these systems are susceptible to intelligent subversion or attacks,” said senior author Radha Poovendran, chair of the UW electrical engineering department and director of the Network Security Lab. “Designing systems that are robust and resilient to adversaries is critical as we move forward in adopting the AI products in everyday applications.”
As can be seen in the following examples, the API wrongly labels a noisy image of a teapot as “biology,” a noisy image of a house as “ecosystem” and a noisy image of an airplane as “bird.” In all cases, the original object is easily recognizable from noisy images.
The fragility of the AI system can have negative consequences. For example, a search engine based on the API may suggest irrelevant images to users, or an image filtering system can be bypassed by adding noise to an image with inappropriate content.
“Such vulnerability of the image analysis system undermines its usability in real-world applications,” said lead author and UW electrical engineering doctoral student Hossein Hosseini. “It’s important to design the system such that it works equally well in adversarial scenarios.”
Researchers are a part of the UW’s Network Security Lab, which was founded Professor Poovendran in 2001. The lab works on the foundations of science and cybersecurity in critical networks. Their research also investigates development robust and resilient systems, like the Google AI, that need to function in adversarial environments for a wide range of applications.
The research is funded by the National Science Foundation, Office of Naval Research and Army Research Office.
More News: